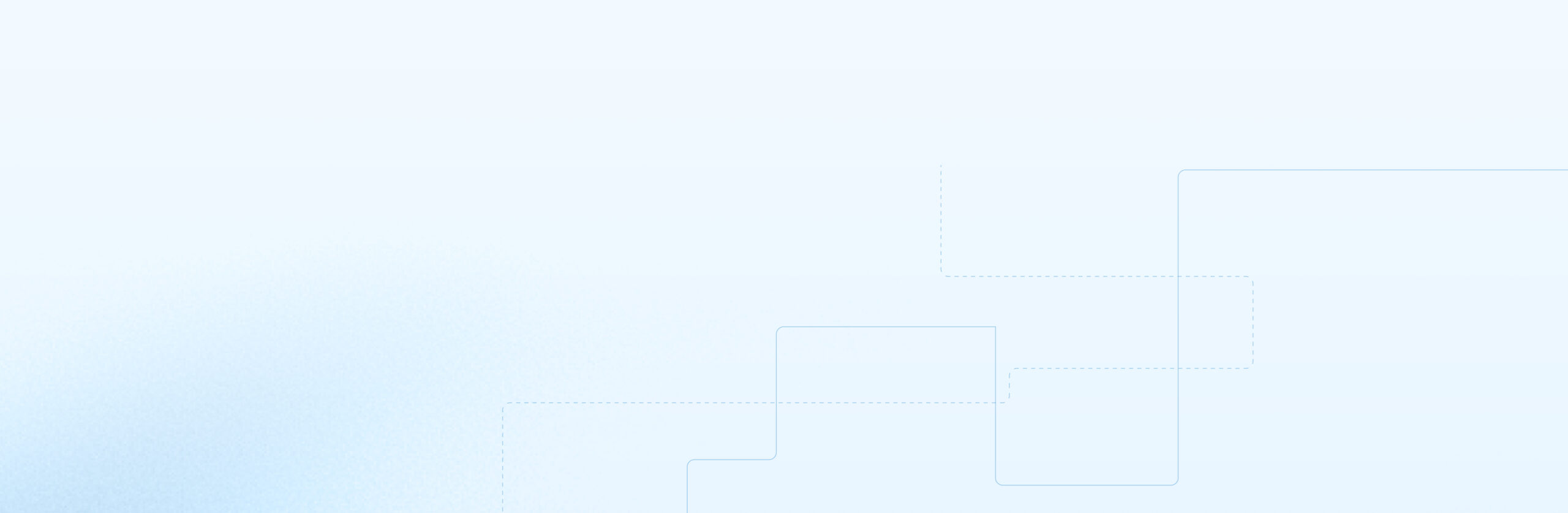
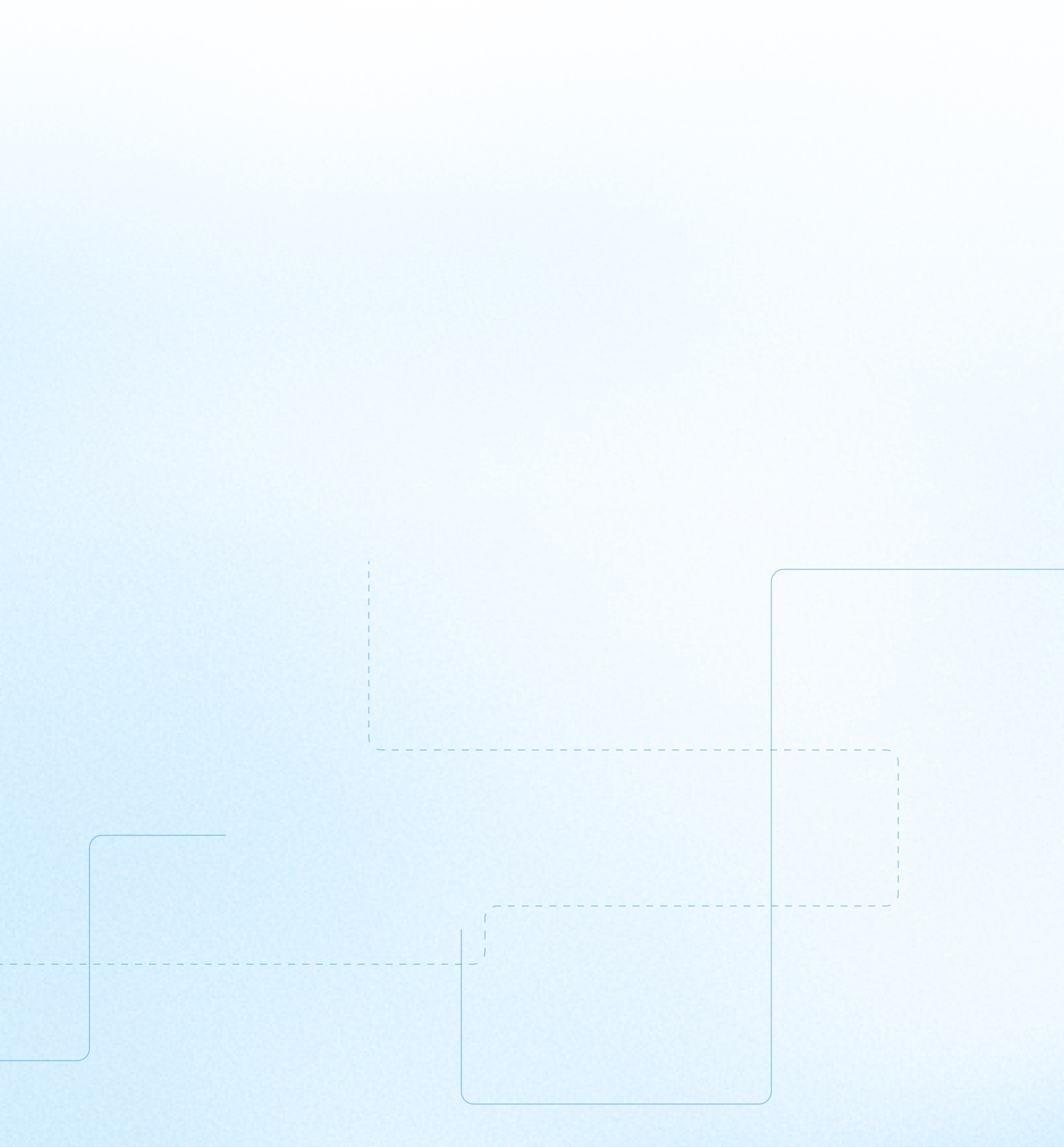
Next Generation
Hybrid Search (Graph + Vector) to Power AI at Scale
Unmatched speed, accuracy, and scalability for advanced AI & ML
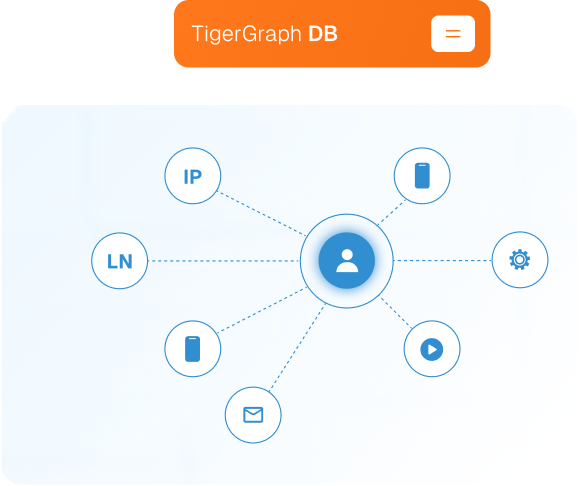
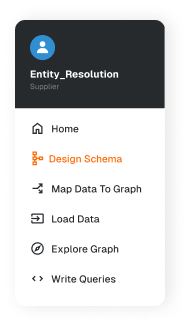
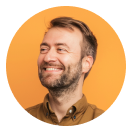
Why Hybrid Search (Graph + Vector)?
AI and ML applications, such as Large Language Models (LLMs) represent complex real-world information using high-dimensional vectors called embeddings. Vector similarity search – finding which objects and concepts are similar – is a foundational part of learning by analogy and making predictions. Businesses use databases with vector similarity search when building their AI and ML applications.
Increasingly, businesses are seeing that the combination of knowledge graphs, graph analytics, and vector similarity search, such as GraphRAG, are the best way to get the most from their data. TigerGraph's multi-modal database is the no-compromise answer for best in class graph and vector data modeling and analytics.
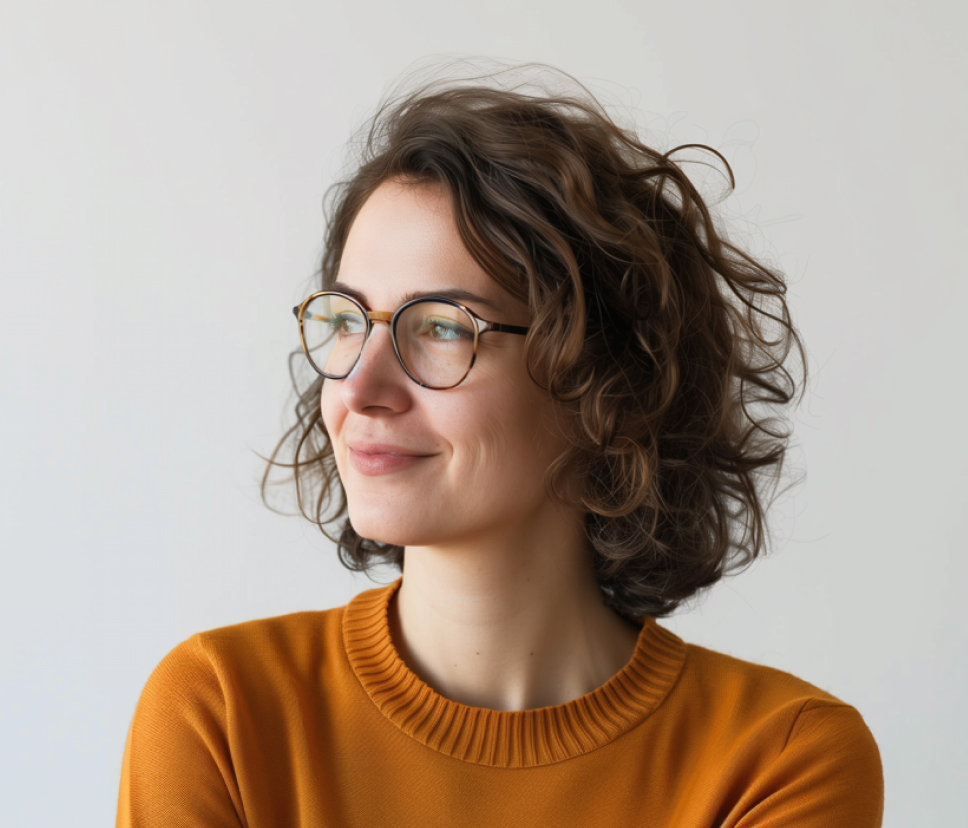
TigerGraph Advantages
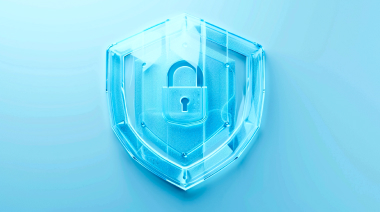
Multi-Modal Graph+Vector
Build sophisticated knowledge graphs with embeddings from structured and unstructured data, for maximum flexibility and expressiveness.
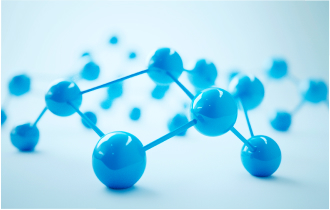
Graph-Enhanced AI
Implement Graph-enhanced AI and ML such as GraphRAG to obtain more accurate and customized AI, with less hallucination and more explainability.
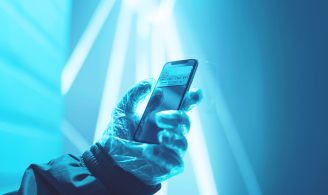
5.2x Faster Vector Searches
With 23% higher recall than any competitors to rapidly uncover the most similar items while using 22.4x fewer resources and reducing operational costs.
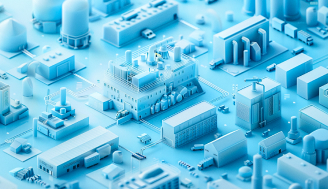
6x Faster Indexing
Blazing fast loading and automatic incremental updating of search indexes, ensuring accurate searches even with large datasets and rapid changes.
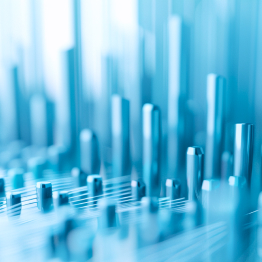
Simpler Operation
Full enterprise-class graph and vector functionality in one database, reducing development and operational effort.
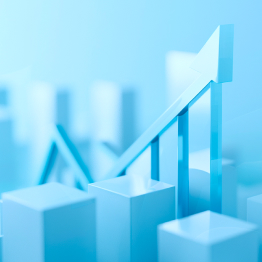
Advanced Hybrid Search
Get answers using graph queries, vector searches, or hybrid searches all within GSQL for enhanced discoverability and contextual understanding.
Success Stories
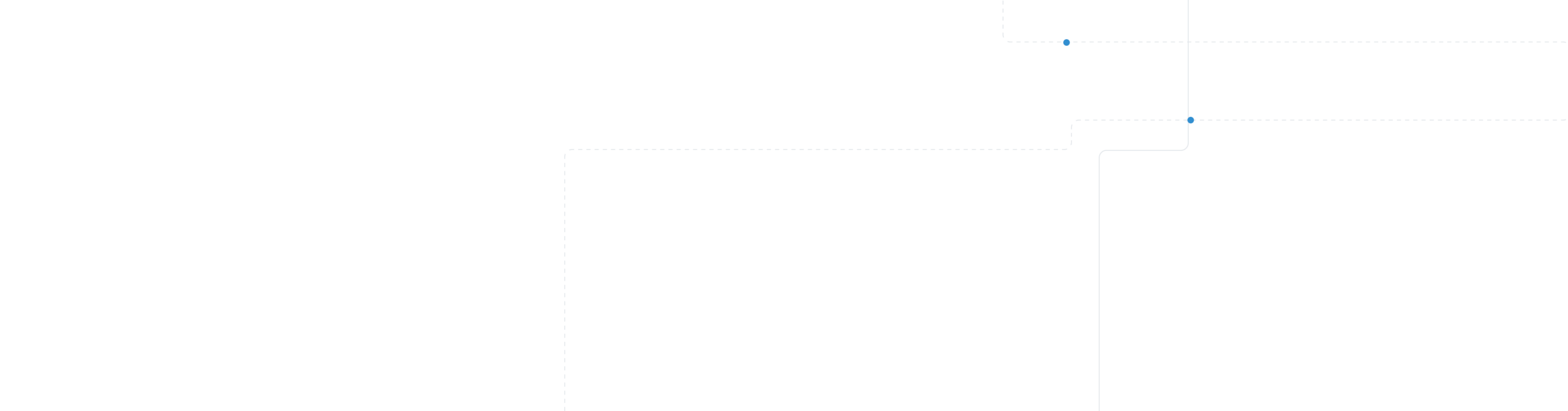
Learn more. Get inspired.
Read Documentation
TigerGraph University
Join the Community
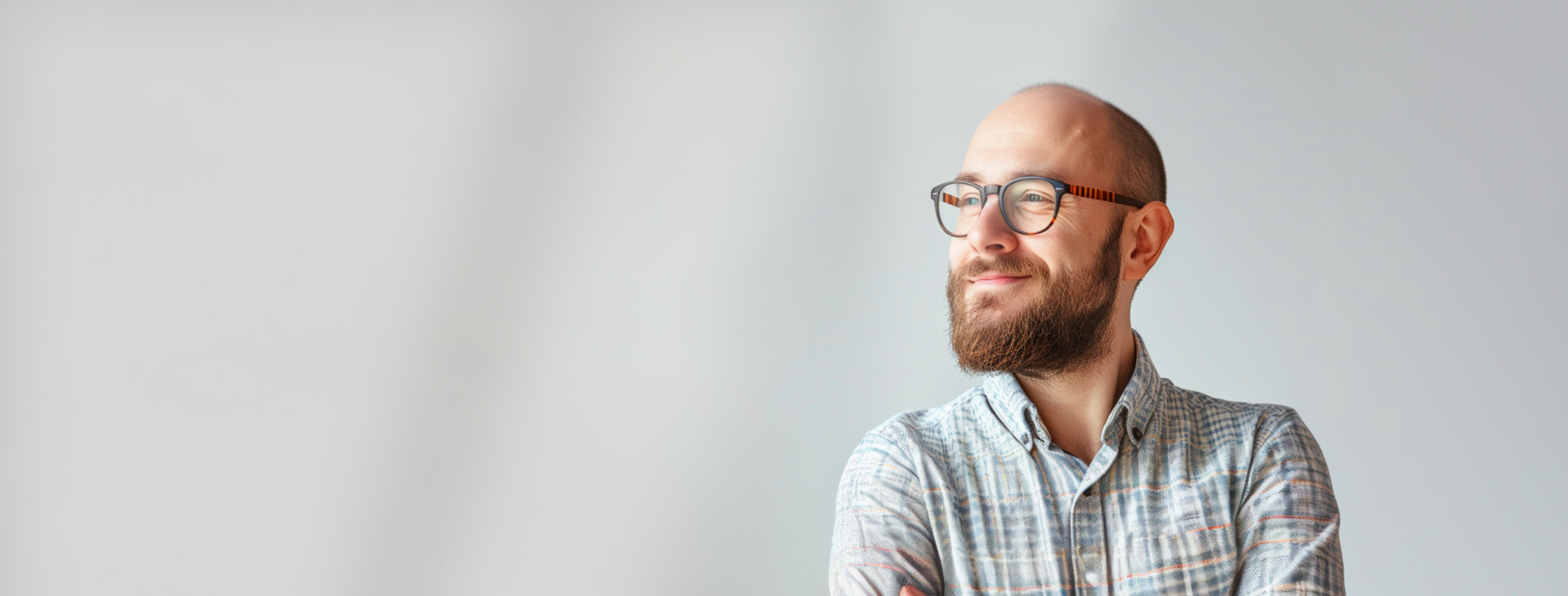